Operators in today’s modern control rooms face serious challenges to understand what’s going on inside their plants as the number of alarms increase. Configuring an alarm has never been easier.
“The amount of alarms is a clear challenge for us today – it is challenging for the operator to understand what’s going on.” -Sr. Advisor IOC (Major E&P company)
What used to be a limited set of well-engineered alarms, built up based on years of experience, has now been replaced by IOT devices and hardware manufacturers that are presenting almost endless alarm options, often without considering the total alarm load on the control room operators.
So why isn’t the alarm working as intended now?
The alarm is often associated with a process variable moving outside the safe operating envelope, and should be initiated early enough for the operator to have time to do the following:
- Recognize the deviation
- Understand the root cause
- Find consequences
- Evaluate operational targets
- Decide whether to act
- Select means and objectives
- Prepare counteractions
- Execute
The above list is challenging for many reasons, and one if the main reasons is that classic alarm systems are based on the assumption that:
one alarm = one cause = one consequence = one action
We all know that this is an oversimplification. One alarm can have many different reasons and end up with a number of outcomes, requiring different mitigations. The alarm system will only support the operators in the detection phase. To counter this, automation vendors develop different tools where each alarm may be given additional help on how to understand root cause, consequence, and mitigation. Again, this is not good enough. To understand why, have a look at the following figures:
One specific transmitter may be capable of detecting one root cause only, but this root cause may develop into a number of different consequences based on other conditions in the plant.
Another specific transmitter may detect several root causes, which may develop into different consequences.
With this type of instrumentation, the whole concept of one alarm, one cause, and one consequence does not work!
For this reason, the common practice of color-coding alarms according to criticality, which is a common practice, is an oversimplification. The same signal may result in consequences of different criticality.
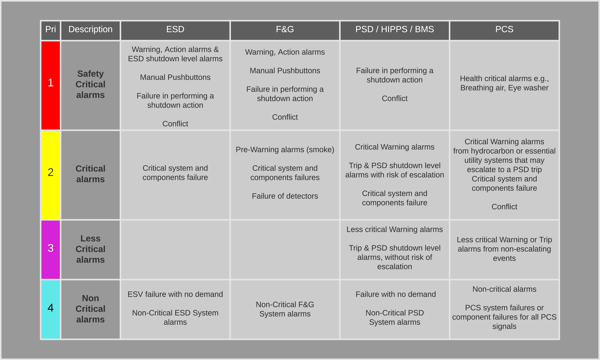
An experienced control room operator will investigate further and look into multiple transmitters, follow timeline trends, and basically use experience before reaching a conclusion on likely root cause and consequence, before initiating any counteraction. The challenge is the number of different alarms and their combinations.
We also know that alarm systems come with major issues such as:
- Alarm flooding - one event triggering a large number of follow-on alarms making it difficult finding the actual root cause
- High alarm rates - number of alarms / time unit
- Standing alarms - always active alarms due to equipment not in operation or other reasons
In order to be able to distinguish between different root causes and different escalation paths, we need to look at all the sensors at the same time.
There will (if correctly instrumented) always be a unique pattern of sensor values needed to identify the root cause. In this way, one could regard the sensors as evidence supporting a theory of what the root cause behind a situation is. Equally, the same pattern can detect how the scenario will develop into consequences.
Please note that this figure is simplified, as one sensor appears to only support one criticality level. There will most likely be a mixture of sensors supporting the different criticality levels.
Possible Mitigations
So how can we improve and help control room operators deal with the alarms triggered from disturbances in these highly complex situations?
Alarm Management
The traditional approach is to work with alarm management, follow up KPI´s on alarm performance, and constantly improve the performance. Techniques such as hiding and shelving, may be introduced. In these cases, rules are made based on operational status of the plant to remove or hide redundant alarms. These, and similar techniques, are actually dealing with the symptoms of alarms not working instead of targeting the root cause behind the problems with alarm systems.
A number of alarm standards and best practices exists, and we recommend the following further reading about this topic: Alarm performance standards by Eldor
Alarm Response Manuals
Another known approach is to add on more information to each single alarm. This is often simple text-based solutions called Alarm Response Manual, Alarm Helper, or similar. Clearly a mitigation strategy, but again trying to combine all possible causes, consequences, and mitigations connected to one alarm is a challenging task and will probably not add much value. Imagine a situation with 5 active alarms, each with an alarm text and an operator with limited time trying to read through all the individual help texts before reaching a conclusion.
A new approach using digital twins
A clearly improved solution would use pattern matching to analyze all the sensors at the same time and further use this insight to conclude on the most likely root cause or even a ranked list of root causes based on the sensor values, ideally with corresponding consequences. This would require a digital twin of the plant where patterns of sensors can be identified.
The figure above shows a mapping of the deviations in the sensor values (blue is low, red is high) against root causes. Such a mapping will be much more accurate detecting specific root causes and consequences.
Artificial intelligence provides a new set of tools for doing this pattern recognition. Two of the most promising approaches are based on machine learning techniques and quantitative physics modelling. The drawback of machine learning is the effort of ensuring the data you’re learning from is correct and manually representative, while it requires limited knowledge about the process. Obviously, this technique will never detect any first unexpected combination of sensors. If you want to detect the first occurrence of an event, machine learning needs to be given some help. Hence, we see the development of hybrid solutions where machine learning and first order models are combined.
With these new approaches the operator can be supported with detecting a situation, finding root causes, understanding the possible consequences with effect on operation, and prepare counteractions. The conclusion is that traditional alarm systems have a number of challenges, but there is hope in digitalization and artificial intelligence. We can now empower the operator with new tools!